Deep Learning – not an Eraser – a new pencil
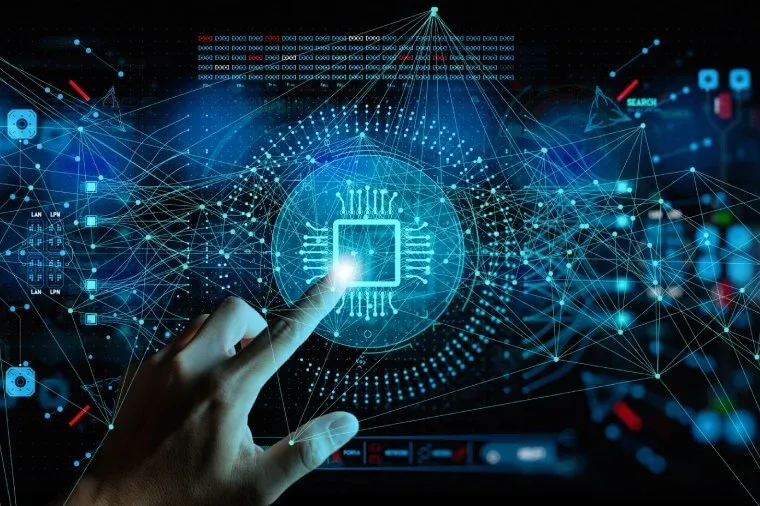
While getting conversant with deep learning tools, remember to get past these top 5 myths.
Deep Learning is neither a bubble nor a rock – and as new and hyped it may be, this technology breakthrough is more like water. It is going to seep slowly and silently into many areas of business, IT and intelligence – making them change in an unprecedented way. But not with a bang. With one drop at a time.
That’s why if you are excited about the new explosion of professional opportunities that deep learning would ignite, you are on the right path. But do not get overwhelmed and over-eager – not just yet. Take a look at these top 5 myths before you decide.
1. First things first, artificial intelligence, machine learning, deep learning and neural networks–they may all sound the same but they are not chops off the same block. Their provenance may be the same but their evolution and application are quite different. So when you are picking a tool to master or a course in any of these areas, do not think that they are interchangeable. A certification in Robotics is not equivalent to being ready for a job in convoluted neural networks. Deep learning is a subset of machine learning. It is not exactly, and all of, machine learning.
2. Secondly, take a jump but not a leap into a different land. The realm is growing at a furious rate with new job and skill requirements. But they are not isolated. In fact, the demand for data science professionals is an interesting upshot of the growth that artificial intelligence is witnessing. If you are already into data mining or database administration, it makes better sense to get proficient in the next level of data science area before considering a course in an entirely new function.
3. Thirdly, deep learning skills may not need a strong level of expertise in mathematics1. Most professionals do not approach this field because they have misconstrued this area as something that needs a Ph.D. level of Mathematics comfort. That may not be true. There is a whole gamut of application and architecture areas of deep learning, where non-mathematical strengths come into play, just as significantly as the ease with numbers. The truth is that any domain of intelligence would need you to be adept at more than one area – like programming, mathematics and statistics.
4. The fourth assumption often congeals around the need for expensive hardware that deep learning training may need. It is not always the case. Today, a host of online tools and cloud options can equip you with the wherewithal that you need to learn deep learning modelling. At the same time, do bear in mind that deep learning is much more than libraries, steps for modelling and functions. It has a lot of dependencies and possibilities that can only be mastered with continuous practice on various models and scenarios. Your problem is not lack of a kit, but the right choice of tools to be picked therein.
5. Do not be disturbed by the fluctuations in the salary levels of some job roles like that of data scientists. Some surveys have sent out alarms that data scientists will find their salaries moving southwards due to an oversupply in the market. The same may happen to deep learning skills someday. But what does not change is the demand for well-rounded professionals. Even if the median salary levels or new job requirements go down a bit (like we saw in 2018 onwards with Dice job postings of Data Scientists), the need for people with both soft and hard skills in a given domain only jumps higher. Add to your baseline of expertise in a continuous and last-mile way. Look at the bigger context of any job you would be doing, master all areas and think long term. Then you would always stay relevant, whether the industry salary average dips or dances.
Note that in 2019, the tools and areas that came up as dominant and positive ones for job listings in this area were these – Python, TensorFlow, PyTorch and even Cloud Platform skills. And as PwC noted, a 2020 estimate can include 2.7 million jobs postings for data science and analytics roles. A recent report from LinkedIn (the 2020 Emerging Jobs Report) also shows that roles like Artificial Intelligence Specialist (which has grown 74% for the last four years), Robotics Engineer (which has seen a 40% spike in demand) and data scientist (with a 37% jump) will lead the top 15 list ahead. But the list still includes specializations like a full-stack engineer, data engineer, cybersecurity professional, cloud engineer and a product owner.
That means that the glacier is just melting down. There is a lot of opportunity for everyone, especially for those who can deliver an overall solution and not just a technology-expert looking for a problem to be solved. Flow in the right stream. It’s going places!
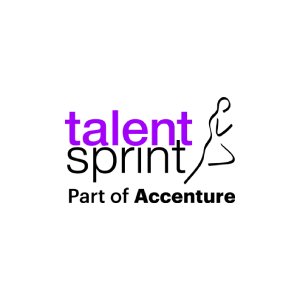
TalentSprint
TalentSprint is a leading deep-tech education company. It partners with esteemed academic institutions and global corporations to offer advanced learning programs in deep-tech, management, and emerging technologies. Known for its high-impact programs co-created with think tanks and experts, TalentSprint blends academic expertise with practical industry experience.