Intelligent Systems – The Next Step in Human Evolution
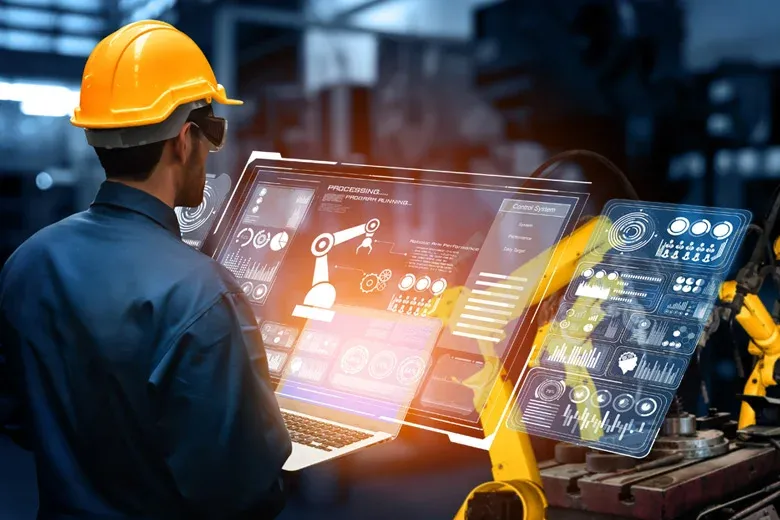
Why did humans discover fire? Why did someone hit the button on the Gutenberg Press? What difference did a wheel make in the journey of mankind? The answer to all such developments from the stone age to the AI age, is captured in one word – augmentation.
Humans need inanimate friends to help them get rid of labor, time, and cognitive effort that can be put in elsewhere or accentuated. Whether a farmer who uses a bullock or a Henry Ford who taps the assembly line- the quest for human augmentation is the same. We want someone to help us amplify the effort we put in. We want someone to help us focus on more important tasks – where creativity, cognitive input, and disruptive ideas matter more. So be it a cow, a piston, or a robot- they are all designed and nurtured for the same purpose- human augmentation. This journey has been accelerated with the onset of intelligent systems.
Let’s face it – nothing augments us as artificial intelligence (AI) does or is capable of doing. An intelligent system is everything packed into one wonder- the convenience of the fire, the wheel’s speed, the scale of the printing press, the loyalty of a farm dog, the precision of an assembly line- and much more.
Intelligent systems transform human lives beyond imagination. Because they are not just about velocity, volume, accuracy, exponential effort, intuitive action, or reliability. They are all of that- and a lot more.
1. What is machine intelligence? What are the types of machine intelligence?
Humans have always been able to tap the agility and horsepower of many other species and tools. But what is different with machine intelligence is that humans can now befriend something that reflects their brain. They have created intelligence beyond their bodies. And poured in smart models, programmable solutions, and clever algorithms. Making their lives easier, faster, deeper, and smarter.
Intelligence comprises many abilities and aspects – comprehension, visualization, processing, memory, reasoning, application, and learning. There are many kinds of intelligence. Here are some broad types:
- Visual / Spatial intelligence
- Linguistic intelligence
- Logical or mathematical intelligence
- Kinesthetic intelligence (aware of body parts and good at physical coordination)
- Naturalistic intelligence (good with environmental areas)
- Intrapersonal intelligence
- Interpersonal intelligence
- Musical intelligence
- Existential intelligence (good at deep questions and philosophy)
When we look at intelligence from the lens of artificial intelligence (AI), there are two main categories- supervised and unsupervised.
In machine learning, models and data help machines to learn. This learning, then, allows machines to support humans in their decisions and actions. When this learning happens without human supervision, it is called unsupervised machine learning. When it happens with humans in the loop, it is called supervised machine learning. And when the machine learns from feedback continuously and continuously improves itself- it uses reinforcement learning.
In supervised learning, both the input and output are defined, and the machine has to learn how to get from input to output. As a result, accuracy and control are high, but it takes too much time, labeling effort, and scalability issues.
In unsupervised learning, the machine must work out patterns and inferences on its own. Of course, this method can be more error-prone than supervised learning, but it is less labor-intensive.
In reinforcement learning, humans can get to insights that even they may not be aware of- provided they can allow machines some time and errors to get there.
Two percent of the respondents use supervised learning in a recent O’Reilly survey, and 67 percent use deep learning.
There are many more divisions possible. For example, suppose a model uses previously-acquired knowledge. It is crystallized, but if it keeps changing and evolving into something better than before- able to apply abstract thinking for new or unfamiliar problems- it becomes fluid intelligence.
Cognitive computing is where sensors and algorithms help machines see and hear to get closer to some human capabilities. When a machine can make decisions based on data and models, this kind of intelligence is called artificial intelligence (AI). Here too, AI can be classified as Narrow (not too many human-like capabilities), General (Matching human capabilities), and Super (capabilities that are better than humans). In deep learning, a machine can mimic the human brain to some extent with its nervous topology and use of neural networks.
So depending on what an organization’s context and needs are, one can go for a specific type of AI model or machine intelligence alternative. For example, narrow and supervised learning would be a good answer for some applications. But for high-stake and high-value applications- one might need reinforcement intelligence and super-AI.
2. How does machine intelligence work?
Now that we know what machine intelligence is composed of let’s say we have decided on one particular course- let’s see if we can get a peek into what happens underneath the lid of these systems. But, of course, that would be wise to do before we unleash the beast in a given direction.
Inside any kind of machine intelligence, a whole new world of modeling and decision trees is brewing. The data and information that goes in or is built up on top of basic inputs get processed and translated in unique ways based on the design and purport of these intelligent machines.
It is all about how algorithms are structured and run – that makes machines churn out so many decisions and insights- and so swiftly. The input and output parameters and the data fed into them in a supervised mode help a machine generate the answers required. In an unsupervised mode, the machine unlocks the patterns and interpretation from all the data fed into it. Then, these help the machine whip up all the answers and actions expected of it. In reinforcement learning, we tap the ability of machines to learn from even bad decisions and past actions. These lessons help them to churn out insights.
As intelligent human beings, we have never left our horses to go wild or our factories to run in the dark – so the same wisdom should apply again with AI. We need to be cognizant of what’s happening inside these intelligent machines. We must maintain visibility, context, responsibility, and far-sightedness while using these mind-blowing wonders.
In the reckoning of Forrester analysts, AI systems making inexplicable decisions can be dangerous. As more and more companies adopt AI, business stakeholders will rely on AI for their workflows. Their trust cannot emerge without a general understanding of how they were made. What is also worth considering here is that AI obfuscates the ‘second-order insights’ like non-intuitive correlations that emerge from the inner workings of a machine-learning model. As advised by Forrester analysts, it would also become necessary for many stakeholders and regulators to understand how the entire model operates. They would need global explanations, while your customers may look for local explanations that clarify how the system made the decision that impacted them.
Also, the quintessential ‘Black Box’ problem of AI is a real challenge when applying machine learning. Even if a machine is spinning out beautiful and thunder-fast answers – one has to know what is going on inside the box that is leading to these answers. It is significant to see the process of helping a machine learn anything. Otherwise, these insights can easily be prone to costly errors, bias, discrimination, false positives, and ethical problems. According to McKinsey’s ‘The State of AI in 2021’ report,
- 57 percent cited cybersecurity as a relevant AI risk. Some companies also report personal and individual privacy as a relevant AI risk more often
- Explainability continues to be an important risk area – whether it is for emerging economies (34 percent) or developed ones (44 percent)
- With AI, these economies also found fairness and equity as significant risk factors (30 percent). What is notable here is that high performers put in effort in managing these risks
- Data professionals actively check for skewed or biased data during data ingestion (47 percent)
- Data professionals actively check for skewed or biased data at several stages of model development (36 percent)
- High performers have a dedicated governance committee that includes risk and legal professionals (23 percent).
3. Voice and language-driven intelligence
Now that we know what happens under the hood of these wonders, let’s spend a few minutes on one of these wonders. It is called voice recognition. And it is extraordinary because it cannot just hear us but listen to us – quite well. It is a genre of AI that can listen, translate, interpret and act in a real-time voice. It can transcribe conversations as they happen. It uses machine intelligence and Natural Language Processing (NLP) for instant speech recognition and contextual translation. It can also distinguish one voice or speaker from another.
In voice intelligence, the solution or model of AI being deployed for a specific case can listen to the voice, record data, process it, and apply it for insights or decisions in an intelligent way. This solution learns from the data it hears. This ability transpires into many solutions like conversational AI, chat-bots, NLP-powered algorithms, and smart assistants. They are permeating all aspects of our lives and are redefining many industries. Today, voice recognition is getting stronger and stronger every day- and is still evolving in many aspects. However, while 89 percent of users say that voice technology is easy to use, they look for better accuracy as the most desired improvement. And 57 percent of users opined that improvements in accuracy would cause them to use voice technology more often or for more purposes. The privacy and comfort parts of this technology also need to get better. This is apparent when 62 percent feel awkward using voice technology when other people are present. That reminds us that while technology embraces maturity and sophistication at a compelling velocity, much more needs to be considered as we augment human power.
Conclusion
Augmentation is a path that is still on an inception curve. As a result, we have barely scratched the surface of intelligent systems’ vast and boundless potential.
Machine learning is exploding in its reach and depth. According to Fortune Business Insights, the global Machine Learning market was valued at $15.44 billion in 2021 and is expected to grow to $21.17 billion by 2022 and $209.91 billion by 2029. Today, AI manifests in many gains and revolutionary impacts – and multiple industries.
As per a recent PwC Global Artificial Intelligence study, there is a $15.7trillion potential contribution to the global economy by 2030 from AI. $6.6 trillion is likely to come from increased productivity, and $9.1 trillion is expected from consumption-side effects. Businesses expect a +26 percent boost in GDP for local economies from AI by 2030. As per this study, Labour productivity improvements would-be drivers of initial GDP gains as firms seek to “augment” their labor force’s productivity with AI technologies and automate some tasks and roles. About 45 percent of total economic gains by 2030 could be coming from product enhancements, stimulating consumer demand. This will translate into a lot of market explosion. Interestingly, worldwide revenues for the AI market, including software, hardware, and services, are forecast to grow 16.4 percent year over year in 2021 to $327.5 billion, as per some estimates from the International Data Corporation (IDC) Worldwide Semi-annual Artificial Intelligence Tracker. By 2024, the market could get past the $500 billion mark with a five-year compound annual growth rate (CAGR) of 17.5 percent and total revenues reaching an impressive $554.3 billion.
Businesses are expecting a lot from AI. A new report published by information hub The AI Journal, titled ‘AI in a Post-COVID-19 World’, has revealed that 72 percent of leaders feel optimistic about the role that AI will play in the future, with the number one expectation being that it will make business processes more efficient (74 percent). About 55 percent suggest that AI will help create new business models, and 54 percent expect it to enable the creation of new products and services. What is notable is that 60 percent of respondents said their organization currently uses AI; 52 percent were planning and implementation. Machine learning is being used in many companies (70 percent), and 63 percent plan further integrations.
There are still issues to be confronted – like a lack of understanding or commitment towards investing at the board level – feared by 59 percent of respondents. Other concerns are the legacy processes and technologies within businesses that do not support AI (50 percent) and the lack of relevant skills within the workforce (48 percent).
Yes, we cannot ignore the execution side and the challenges around the adoption of intelligent systems. In a recent O’Reilly survey on AI, it was spotted that the most significant barrier to AI adoption is the lack of skilled people and the difficulty of hiring. There are also challenges to the availability of quality data. Organizations struggle with a lack of skilled people and difficulty hiring (19 percent) and data quality (18 percent). There are huge skills gaps in areas like ML modelers and data scientists (52 percent), understanding business use cases (49 percent), and data engineering (42 percent). People are also dealing with Interpretability, model degradation over time, privacy, and fairness (50 percent), security as a concern (42 percent) – as seen in the O’Reilly survey.
AI-ready talent and skills – are critical precursors before we can start dreaming about reaching unprecedented business goals with machine learning and AI. According to Deloitte’s Tech trends 2021 report, MLOps can help AI teams promote trust by addressing data management challenges such as accountability and transparency, regulation and compliance, and ethics. Also, multi-talented teams of technologists and machine learning professionals can help organizations operationalize and scale AI. To IDC’s estimates, 28 percent of AI/machine learning projects fail due to a lack of necessary expertise, production-ready data, and integrated development environments. In addition, about 47 percent fail to make it out of the experimental phase and into production.
The question, now, is – how do we keep these intelligent systems as much in control as we want. The worry now is- can we tame them the way we domesticated fire, horses, light bulbs, engines, and even space-crafts? The challenge now is- can we squeeze the most out of them without losing anything from our side? Do we have the right knowledge buttons and skills to drive these augmentative forces? That’s the real test of human augmentation. And the ultimate sign of intelligence. Human intelligence.
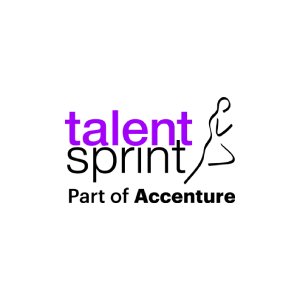
TalentSprint
TalentSprint is a leading deep-tech education company. It partners with esteemed academic institutions and global corporations to offer advanced learning programs in deep-tech, management, and emerging technologies. Known for its high-impact programs co-created with think tanks and experts, TalentSprint blends academic expertise with practical industry experience.