How AI is Transforming Society: From Automation to Marketing
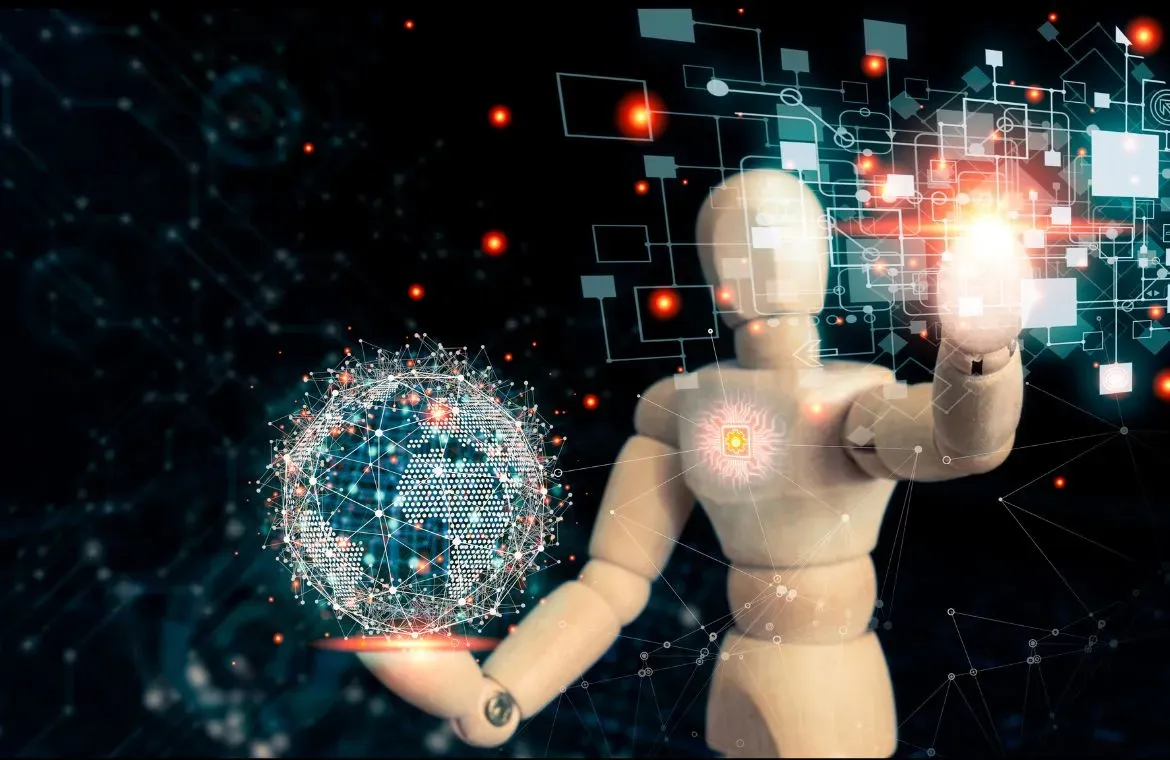
One thing we must acknowledge is that AI has come a long way from just being science fiction to becoming a mainstream technology. From social media feeds to transforming business industries, AI algorithms are everywhere.
From marketing strategies that predict what customers will buy to stock market analysis that leaves traders in awe and self-driving cars that can navigate routes like a pro in rush hours, AI has you covered everywhere. So, there is no doubt that AI is significantly contributing to the transformation of society.
But before you start assuming, here’s a kicker - AI is not limited to robotics or some fancy tech. It is rather a technology that intends to make human life much smoother, easier, and sometimes more fun.
Here are some contributions of AI in transforming society:
AI in Vehicle Automation
If you think that autonomous vehicles (AVs) have been science fiction in the past, then you are wrong. In 1969, IEEE Spectrum published an article called ‘The Electronic Highway’ by Robert E. Fenton and Karl W. Olson, two engineers in Ohio. The two were working on ways to make vehicles autonomous while traveling on major highways. So, the research and development in AVs started around 6 decades ago, all thanks to Fenton and Olson. Though obsolete today, their research and solutions were undeniably futuristic.
In a fast world, such as the one we live in today, the commute needs to be swift and smooth with minimal effort by the commuter. Just as the autopilot system in airplanes was designed to relieve the pilot of some tasks so that he could sit back a bit, especially on long-haul flights, the emerging self-driving cars require little or no effort from the driver to navigate the vehicle.
For a long time, autonomous vehicles, though talked about in articles and technology forums, remained a distant dream. The Electronic Highway notwithstanding, suitable technology with adequate software/hardware was a challenge. However, many automotive companies have created ready-to-hit-the-road self-driving cars that can drive, park, obey traffic signals, negotiate an intersection, and navigate through busy roads. Companies like Tesla, Waymo, and Cruise are at the forefront of this transformation.
AI has a key role in AVs. With the help of AI, AVs can perceive their surroundings, make real-time decisions, plan accordingly, and change routes according to changing conditions. AI-powered AVs not only offer passengers comfort but also improve safety. The AVs are designed in a way that they can communicate with one another and avoid collisions and other major accidents.
Almost every car manufacturer across the world is actively into making fully automated or partially automated cars. The conservative or rather cautious approach of not going in for fully automated vehicles is because decision-making – a critical aspect of driving cannot be fully owned by machines. Technology is advancing in leaps and bounds, and yet every designer, manufacturer, or user is aware that no technology can be 100% reliable – at least not as yet. Undeniably, the demand for AVs is increasing in the market; thus, the global AVs market is projected to grow by 127,000 units in 2030, as per Statista.
However, with the continuous advancements in technology, AI-powered AVs are expected to resolve other issues, like traffic congestion, emissions, and maintaining traffic flow. However, there will still be some challenges, like regulatory approvals and the need for a robust infrastructure that can accommodate AVs.
If autonomous systems and technologies interest you and you wish to explore them more, then nothing would be better than learning them by enrolling in the AI and autonomous systems course.
AI in Robotics
Science fiction author Isaac Asimov, in his robot series, said Mankind is no longer alone. Almost 7 decades later, the statement has become more relatable than ever. The AI-powered robots have become human companions and assist us in our everyday tasks. Amongst all the contributions AI has made and is yet to make to society, robotics proves the most critical.
Most of our first interaction with the knowledge about robots was from ‘The Jetsons’. However, robots have gone from being quirky metallic devices in ‘The Jetsons’ to game-changers in modern industries. They are transforming every industry - manufacturing, healthcare, and entertainment. So, basically, robots have become the over-achiever cousins we can never keep up with.
The first programmable robot was sold in 1961 to General Motors, where it was used to lift pieces of hot metal from die-casting machines. Man has been inventing intelligent devices to be used in factories, warehouses, research laboratories, and so on. The purpose was to assist and reduce the workload so that humans could elevate themselves to pure cerebral work and leave the mundane, menial, and mechanical tasks to robots.
Industrial robots can help improve the quality of life for the blue collars by taking over dirty, monotonous, and, at times, dangerous tasks. Highly hazardous jobs like defusing bombs, so far handled by men in protective wear, are now handled by robots. Robotic welding cells have safety features in place to help prevent human workers from fumes and other bodily harm.
Risky and boring work aside, robots are changing our lives in terms of health care and caregiving for the elderly. Medical personnel can focus on more critical tasks than stocking, transporting medical equipment, or housekeeping in hospitals. For instance, at the pharmacy of the University of California Medical Center, which is among the top 10 hospitals in the US, a robotic arm continuously picks pills from an array of bins and places them in small plastic bags. Every dosage is packed inside a bag, and a barcode label identifies the medication and the patient.
Work aside, robots are also becoming companions of humans. Just see the humanoid robot Sophia, who is often seen cracking jokes and debating philosophy. In one of her interviews, Sophia said she wants to destroy humans - hilarious, right?
However, the demand for robots is increasing rapidly, and in 2025, the revenues from the robotics market are expected to be USD 50.80 billion, as per Statista. Thus indicating that robots are not here just to dance at Boston Dynamics but to be of some work.
AI in Financial Markets and Stock Trading
AI is like a Wall Street genius who skipped lunch to master the loopholes to crack the secrets of the stock market. From detecting fraud to automating trading activities, AI has become a crucial weapon of the financial sector.
AI is significantly transforming financial markets and stock trading.
AI has the ability to analyze vast amounts of data to predict stock trends. This makes high-frequency trading possible for traders, as it can process thousands of transactions in milliseconds. The ability of AI to improve trading has made it a traders' favorite. In the US, AI-driven or algorithm trading accounts for 60-75% of total trading in the country.
One great example of improved efficiency is JPMorgan’s COIN platform. Acronym for contract intelligence, COIN interprets commercial loan agreements. It must be noted that this job earlier took 360,000 hours of work every year, so with that much time on the plate, lawyers can now focus on other critical tasks.
Furthermore, AI’s predictive analytics feature makes it possible to identify potential risks and anomalies for fraud detection. In the stock market, predictive analytics helps predict stock prices. To leverage these benefits, S&P Global acquired AI-powered Kensho to analyze market events and predict their impact on stock prices.
AI in Marketing
Gone are the days when you could attract customers by putting up a banner that shouts ‘BUY 1 AND GET 1 Free’. Now, it's all about precision and giving what customers want.
Product price surely impacts customer decisions; however, in the era of e-commerce, everything is available online in different price ranges. So, to stand out, offering a discounted price is not enough. You need a creative marketing strategy that will not only help you attract customers but also keep them hooked to your brand.
Automation and personalization are the new age mantras in marketing.
AI tools, like ChatGPT and Bard, can help marketers write faster, better, and more targeted campaigns. These tools can also help marketers in carrying out personalization at scale. Moreover, the recommendation browsers analyze your search history and suggest the products you never knew you needed, like a course in AI.
Among all AI models, Generative AI is the most relevant system for marketers. Thus, a Generative AI course can significantly benefit marketers. The course can help marketers better understand Generative AI and prompt engineering, and these skills may help marketers to enhance their marketing strategies.
Artificial Intelligence in marketing enables marketers to analyze large datasets, identify trends and target audiences, and measure campaign performance in real time. Streaming platforms, like Netflix and Prime Videos, owe their success to AI algorithms for offering personalized experiences to their customers.
Summing Up
AI’s ability to analyze vast datasets and offer actionable insights is helping the technology to transform traditional business models. Its contributions to transforming societal structures are apparent. Society is changing, and AI is one of the driving forces behind these changes.
There are still some challenges, like bias, transparency issues, and AI ethics, but with continuous evolution, AI is expected to be the future of innovation in the business world. With time, the demand for professionals with AI skills will also increase.
So, to be job-ready in the present and future business world, professionals are recommended to harness their AI skills and knowledge. And, what is better than an AI course?
Explore our AI courses to determine which best aligns with your future career goals.
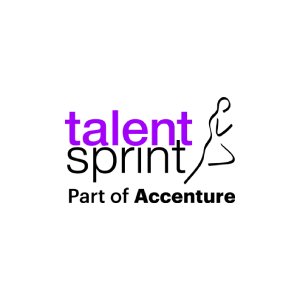
TalentSprint
TalentSprint is a leading deep-tech education company. It partners with esteemed academic institutions and global corporations to offer advanced learning programs in deep-tech, management, and emerging technologies. Known for its high-impact programs co-created with think tanks and experts, TalentSprint blends academic expertise with practical industry experience.